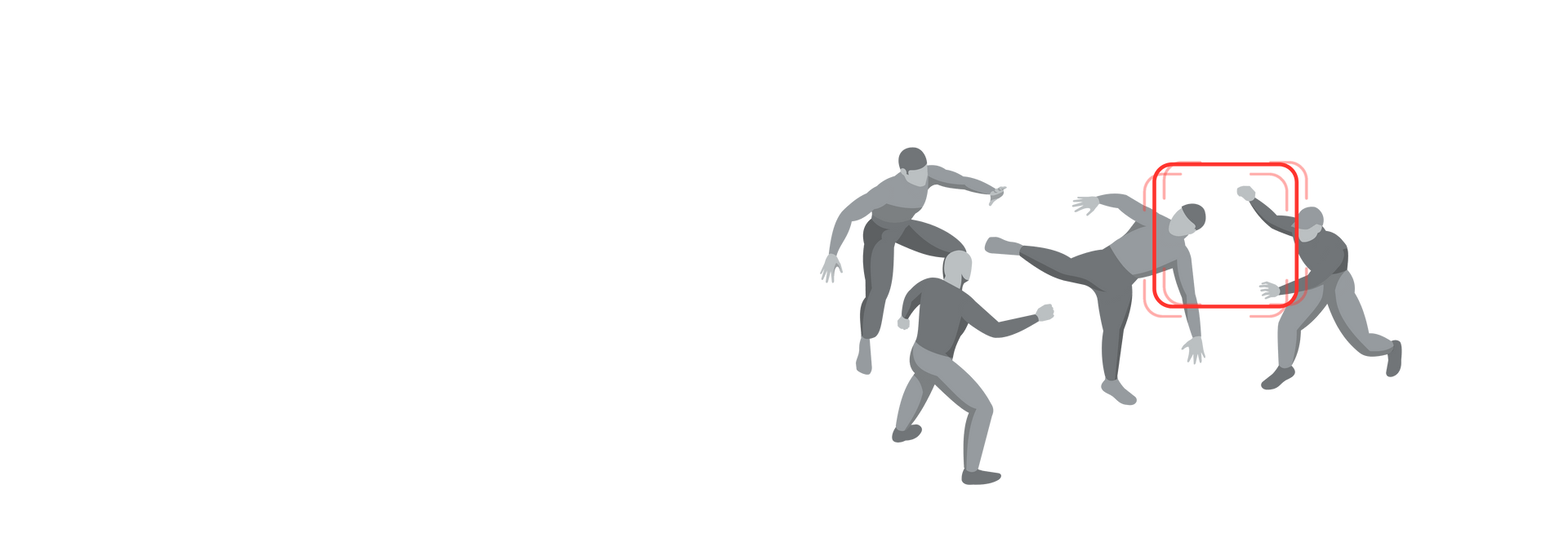
Anomaly Detection and Behavior Recognition
Scylla Anomaly Detection and Behavior Recognition allows security professionals to detect and identify high-impact anomalous events as they occur.
Book a demoRelated materials
FAQ
There are 3 submodules of the Scylla Anomaly Detection and Behavior Recognition system, including a fight and violence detection module, smoke and fire detection module, and a module that detects suspicious shopping behavior that can result in shoplifting. All three Scylla Anomaly Detection and Behavior Recognition submodules analyze such events from CCTV video footage either in real-time or forensically, from video databases. When a specific event is detected, the system initiates a corresponding alert and distributes it through predefined alerting pathways to the end-user.
A sequence of frames is provided to the pre-trained neural network engine of Scylla Anomaly Detection and Behavior Recognition. Thus this 3D matrix (2D frames + time) is considered as an input. Scylla Anomaly Detection and Behavior Recognition AI then decides if the actions in this matrix correspond to the action sought after and triggers an alert if the probability is above the defined threshold.
The anomalies detected by Scylla Anomaly Detection and Behavior Recognition are based on the training set. In particular, the fight detection model is trained on a large real-world dataset of fights and assault cases recorded from CCTV cameras. Thus the anomaly it detects will include acts of violence including fighting and wrestling. Similarly, the smoke & fire detection submodule of Scylla Anomaly Detection and Behavior Recognition is triggered when these hazardous events are detected in the scenery.
The system accumulates several consecutive frames and analyses the whole batch as a unit. The duration of this batch is different for different submodules but on average the chunk duration is 3-5 seconds.
The maximal distance will depend on the camera characteristics. More specifically, on the lens. Typically, for proper illumination (400 lx and higher) the requirement is that the person height should take up 1/6th of the frame height. For most cameras this results in maximal detection distances of up to 15 meters. If optical or digital zoom is used or the camera doesn't have a standard aperture/focal range, the maximal distance can vary.
Absolutely. After all, what Scylla Anomaly Detection and Behavior Recognition needs is the video feed from CCTV which is provided by most CCTV networks and video management systems. Moreover, Scylla Anomaly Detection and Behavior Recognition can be two-way integrated with major VMS providers, such as Milestone, Genetec, Network Optix, and others.
An alert containing all the crucial information is compiled and delivered to end-users responsible for security. There are several customizable alerting pathways: Scylla dashboard, Scylla mobile alerting application, access point relay boards, and VMS alerting API, to name a few.
The shoplifting detection model is trained on a large dataset of videos from surveillance cameras, where shoplifting events occur. The submodule is designed to detect an action of taking an item and trying to conceal it in a bag or backpack. The system is supposed to analyze the streams coming from the retail environment excluding the cashier area since after paying for an item a customer could take actions that are identical to concealing goods. Please note that since shoplifting itself is a subtle event to accurately detect with a high probability, Scylla monitors suspicious behavior that can result in shoplifting.
Yes. The alert is triggered based on the actions in the camera view. Facial biometrics plays no role in the decision making process of AI.
Yes, Scylla Anomaly Detection and Behavior Recognition can work both on cloud and on premise.
Scylla Anomaly Detection and Behavior Recognition is designed to help security units by supporting their daily operations, augmenting their capabilities and eliminating possible human-factor related flaws. Also, in case of a possible threat the alert that is sent out by Scylla is enriched with information crucial for quick and inclusive analysis of the threat on site and effective planning of dedicated counteractions.
Smoke & fire detection submodule is built on the largest dataset for the Anomaly Detection and Behavior Recognition models so far. As the title suggests, the system alerts in the event of either smoke or fire. The environment where the system could be installed can be both indoors and outdoors.
Scylla Anomaly Detection and Behavior Recognition treats the whole frame and analyses all actions within the frame. Thus, regardless of the number of fighting instances in the frame - the alert will be triggered if there are any instances of violence.
The acts of fights and vandalism are detected by a dedicated model that was trained on a large amount of real-world video recordings from CCTV cameras. The model for suspicious shopping behavior detection is separate and it is trained to detect possible shoplifting actions only.