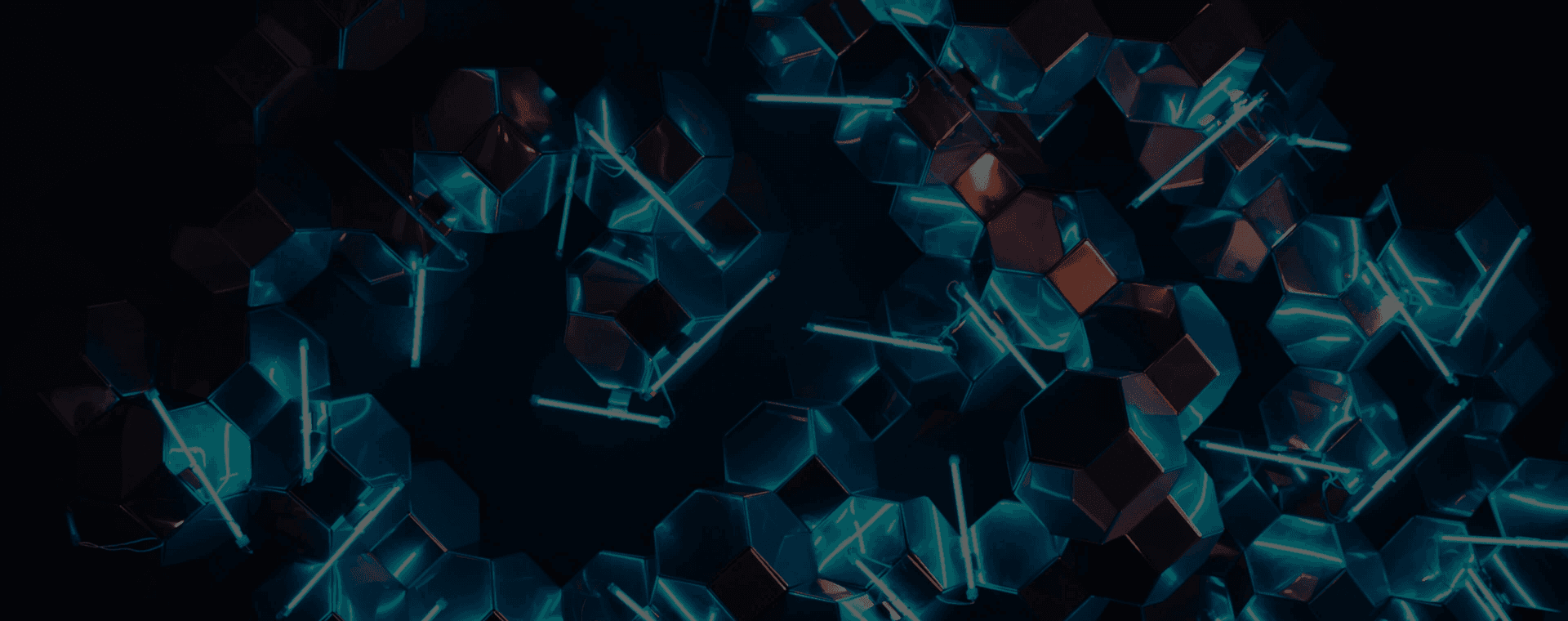
Video Analytics Trends Part 3: Composite Detections

Graeme Woods
Global Business Analyst
Welcome to part three of a five-part series on trends in video analytics.
Last time, I discussed how AI will become increasingly important in drawing insights by using both unstructured data, such as video, and structured data such as ERP solutions.
This time, we’re covering the trend towards analytics that combine (or composite) multiple detection types in both time and spatial dimensions (spatiotemporal) to provide more useful and accurate analytics.
Legacy solutions use only one simple detection. For example, motion detection, which simply measures the number of pixels changing within the specified part of the camera view and sends an alert if a certain proportion of pixels change.
The problem with this very simple analytic is pixel changes are not always related to something that we are interested in. Most of the time, the pixels change because of something like leaves moving in the wind, a random headlight shining in, moths or pets or camera issues. This means that the rate of false positives will be very high, making this almost useless as a detection and it certainly can’t be used to produce an actionable insight.
Unsophisticated, simple detections are often bundled with cameras or included with VMS software at a low price. This low cost reflects their minimal business benefits compared to more sophisticated solutions like Scylla that are cost-effective and bring substantial benefits and ROI.
Scylla starts with motion detection but makes it useful by adding person and vehicle detection. This virtually eliminates all false positives, so there will only be an alarm if there is movement, and the movement is caused by a person or vehicle entering a restricted area.
Scylla’s analytics products are largely differentiated through composite detections. An example of this type is Scylla’s suspicious shopping behavior analytics which combines object detection and action detection algorithms to identify suspicious shopping behavior that can result in shoplifting.
Whilst a simple gun detection algorithm may simply detect something that looks like a gun, a more advanced algorithm such as Scylla’s active shooter detection combines object identification (detection of a weapon) with action recognition (movement of a person through space over time).
If a child leaves their toy pink water pistol on a table or carries a pencil case that looks like a gun, there is no alarm. Similarly, a guard with a holstered side-arm is not detected. When an armed person pulls out their gun and moves into a shooting stance, then the weapon and the person’s movement detections are combined to identify an active shooting situation.
A simple slip and fall detection algorithm will only find a person-shaped object that is horizontal, while an advanced algorithm will be able to detect the motion of someone slipping and falling, which is a far more accurate and useful detection.
To summarize to date: though very simple analytics such as motion detection are still common in the marketplace, object detection or simple face detection solutions comprise the mainstream market. Many video analytics vendors are still selling these simple solutions, although these will increasingly be given away with camera or VMS purchases.
The current trend is towards integrating several discrete detections to build advanced composite analytics. These offer increased accuracy and business benefits to end users, and this is the path being followed by Scylla.
This is illustrated in the following diagram:
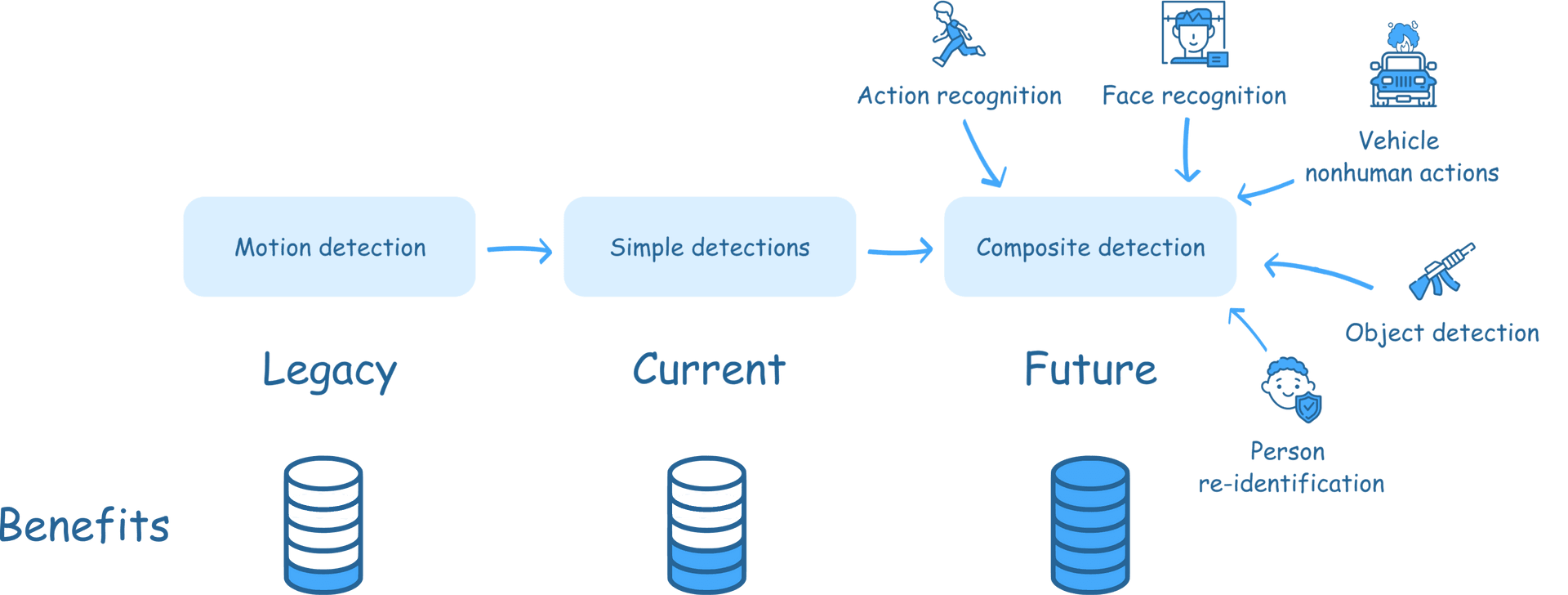
Advanced analytics may combine:
â—Ź Object detection (of a weapon, product or a person)
â—Ź Action recognition of one or more people and their interactions (separately and together)
â—Ź Detection of non-human activities (such as fire / smoke or vehicle motion)
â—Ź Detection of special events using thermal imagery
â—Ź Face recognition to identify individuals
â—Ź Individual recognition based on physical appearance (height, gait) or clothing (can be combined with face recognition)
â—Ź Overlay of human motion over a space (for example, occupancy counting over time or motion through a retail space)
In conclusion, comprising combinations mentioned above, advanced composite analytics offers significant benefits to buyers because of the far greater accuracy and specific detections that are matched to business problems.
More Video Analytics Trends:
Part 1: Combining Structured and Unstructured Data
Part 3: Composite Detections
Stay up to date with all of new stories
Scylla Technologies Inc needs the contact information you provide to us to contact you about our products and services. You may unsubscribe from these communications at any time. For information on how to unsubscribe, as well as our privacy practices and commitment to protecting your privacy, please review our Privacy Policy.
Related materials
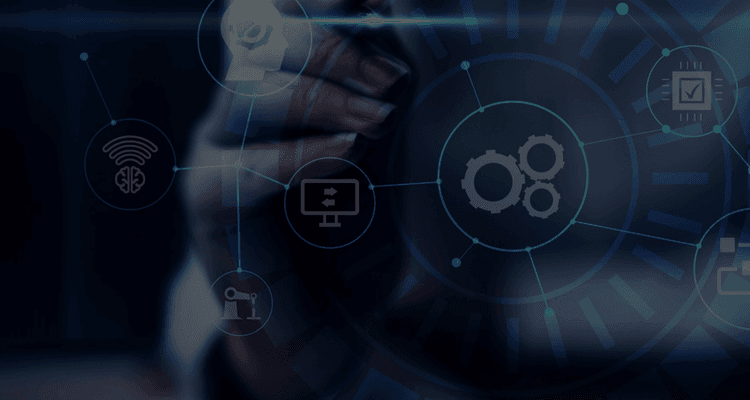
Advantages of Using Artificial Intelligence in Video Surveillance
AI video analytics has gained immense popularity over the past couple of years due to a large number of real-world use cases. Learn about the actual advantages of leveraging AI-powered face recognition, object and intrusion detection solutions for security purposes.
Read more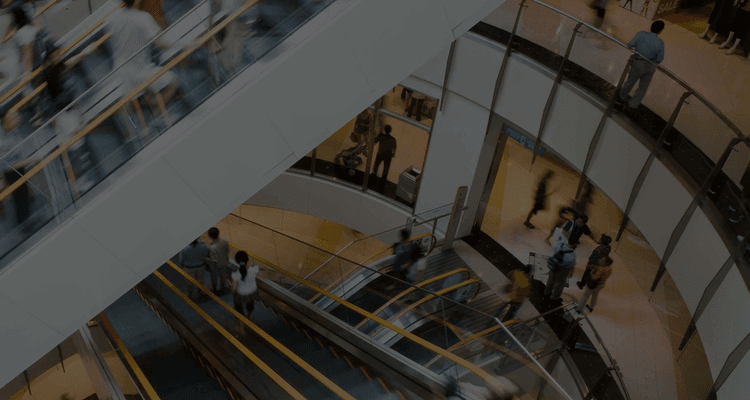
How Artificial Intelligence Can Benefit Retail Security
Updating existing security systems with AI solutions is critical for retailers. Learn how it helps to prevent losses, better understand the security landscape and respond to threats more effectively.
Read more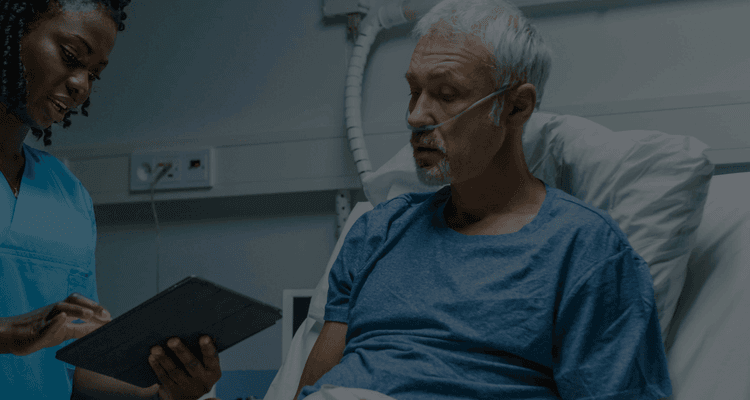
7 Reasons to Implement AI Video Analytics in Healthcare Facilities
Learn how hospitals equipped with AI-powered video analytics bolster their security measures, enhance patient and personnel safety and even optimize their operational efficiency.
Read more