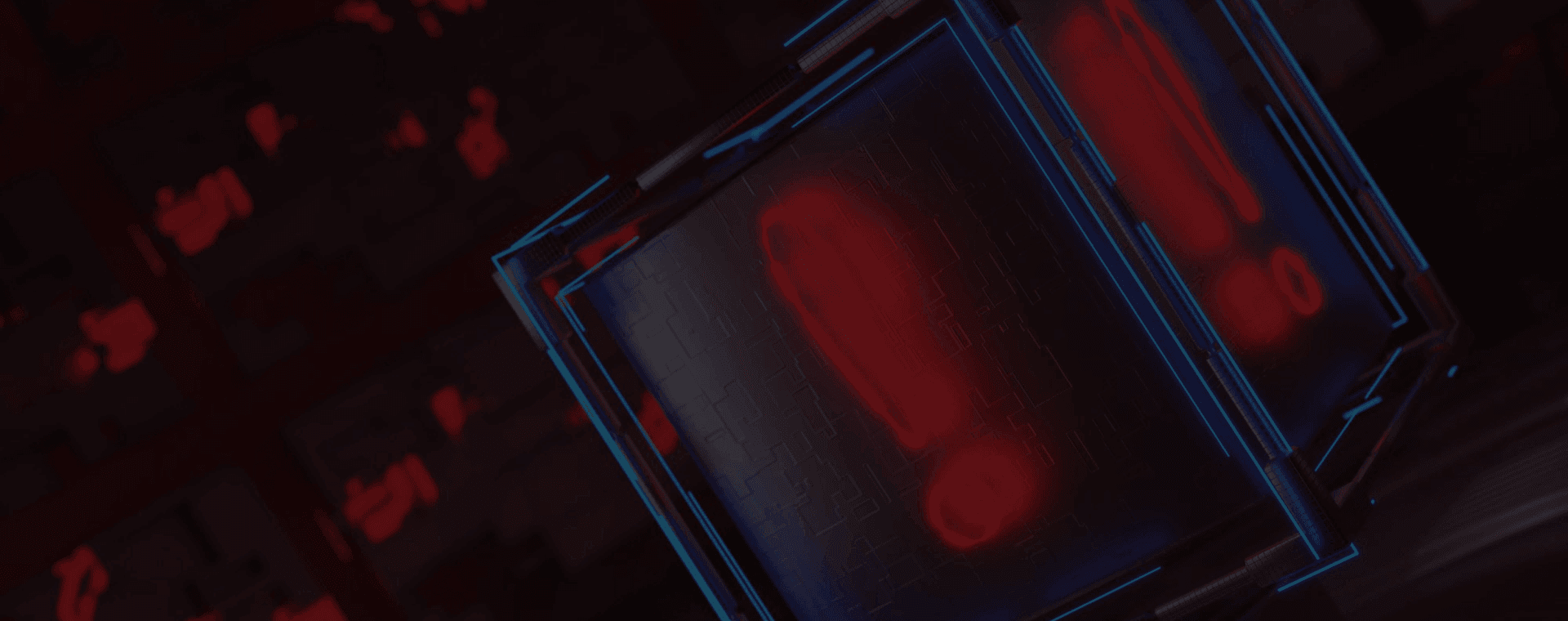
Where False Alarms Come From

Radosław Dzik
PhD, Global Channel Partner Manager | Head of Sales Europe
When it comes to the use of artificial intelligence in physical security, one of the most frequently asked questions is about the false positive rate. Where do the false positives come from? Is it possible to reduce their number? In this article, I’ll give a quick analysis of the issue.
False positives in AI classifiers (analytics) can arise from various sources. Here are some common factors that can contribute to false positive errors.
Imperfect training data: AI classifiers rely on training data to learn patterns and make predictions. If the training data is incomplete, biased, or contains errors, the classifier may produce false positives. For example, if the training data predominantly consists of certain classes or lacks representation of certain cases, the classifier may overgeneralize and produce false positives in underrepresented classes.
Class imbalance: Class imbalance refers to situations where the number of instances in different classes is significantly uneven. If the positive class is underrepresented compared to the negative class, the classifier may have a bias towards predicting the negative class, resulting in false positives for the positive class.

Comment: These two factors are the most common factors in AI used in intrusion (or any object) detection. Neural networks that are supposed to classify humans are trained only with one class examples, that is, to recognize the condition: either human or not. In Scylla, neural network feeding is training as many as 80 different classes, such as different animals, flags, trees and others. This results in a balanced dataset and no bias in classification, and thus a low false alarm rate. Scylla AI video analytics triggers alerts only in case of validated threats, impressively minimizing false positives by up to 99.95%.
Feature representation: The features used by the classifier to make predictions may not adequately capture the underlying patterns in the data. If important features are missing or irrelevant features are included, the classifier might misinterpret the data and generate false positives.

Comment: In Scylla, we take images/video from CCTV cameras for learning, which is exactly the kind of views that analytics will analyze in the future. This also contributes to the low false alarm rate.
Overfitting: Overfitting occurs when a classifier becomes overly specialized to the training data and fails to generalize well to new, unseen examples. In such cases, the classifier may classify outliers or noise in the data as positive instances, leading to false positives.
Inadequate threshold setting: Classifiers often require a threshold to decide whether an instance belongs to a particular class. If the threshold is set too low, the classifier becomes more sensitive and may produce more false positives. Adjusting the threshold can help balance false positives and false negatives, depending on the specific application.
Inherent complexity of the problem: Some classification tasks are inherently challenging, and distinguishing between positive and negative instances may be difficult due to subtle or ambiguous patterns in the data. In such cases, AI classifiers may struggle to achieve high accuracy, leading to false positive errors.
Final Takeaway
Reducing false positives often involves improving the quality and diversity of training data, carefully selecting relevant features, addressing class imbalance, optimizing the classifier's parameters, and fine-tuning the threshold to align with the desired trade-off between false positives and false negatives. So why not use a solution that, at the time of design, already circumvents these problems.
Stay up to date with all of new stories
Scylla Technologies Inc needs the contact information you provide to us to contact you about our products and services. You may unsubscribe from these communications at any time. For information on how to unsubscribe, as well as our privacy practices and commitment to protecting your privacy, please review our Privacy Policy.
Related materials
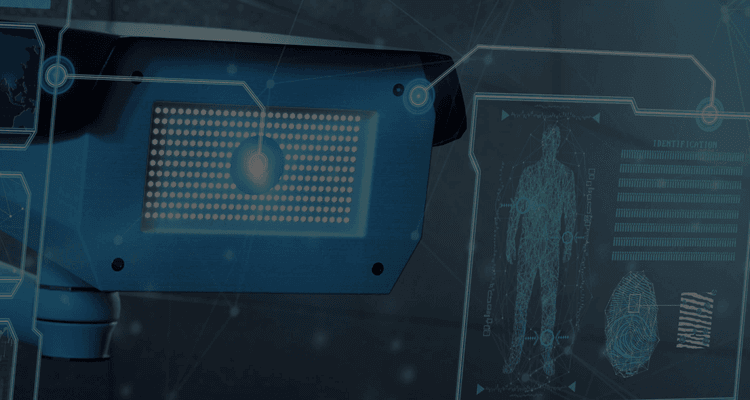
How to Choose the Right Video AI Analytics Supplier
As the global market for AI-based video analytics continues to grow, so does the number of video analytics solution providers. Go through this checklist to choose the right one for you.
Read more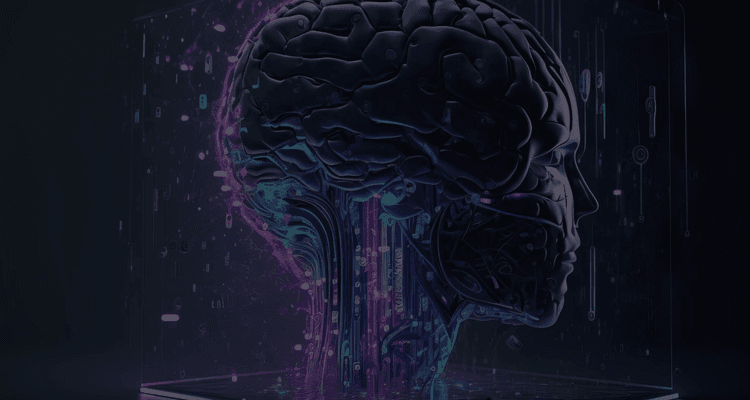
Is any regulation of AI in EU needed? It's coming...
The regulation of AI use is still in its early stages, but there are several legal frameworks and initiatives aimed at addressing the ethical concerns associated with AI.
Read more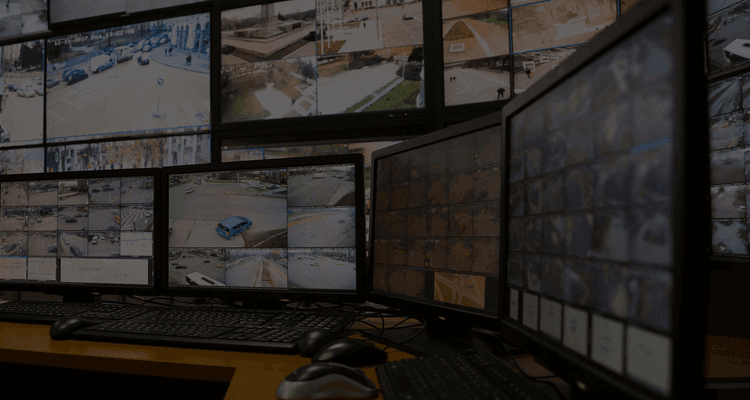
How AI Video Analytics Help Video Monitoring Centers Save Money
Learn how implementing AI-powered analytics in video monitoring centers brings cost savings and a quick return on investment.
Read more